Serviços Personalizados
Journal
Artigo
Indicadores
-
Citado por SciELO
Links relacionados
-
Similares em SciELO
Compartilhar
Revista argentina de cardiología
versão On-line ISSN 1850-3748
Rev. argent. cardiol. vol.92 no.1 Ciudad Autónoma de Buenos Aires mar. 2024 Epub 28-Fev-2024
http://dx.doi.org/10.7775/rac.es.v92.i1.20727
REVIEW ARTICLE
Artificial Intelligence in Cardiovascular Imaging and Cardiovascular Prevention
1Department of Cardiology . Hospital Italiano de Buenos Aires
2Department of Diagnostic Imaging , Hospital Italiano de Buenos Aires
3Department of Health Informatics , Hospital Italiano de Buenos Aires
4Universidad Tecnológica Nacional, Ciudad Autónoma de Buenos Aires, Argentina
5Department of Medical Informatics , Instituto Universitario del Hospital Italiano de Buenos Aires
Artificial intelligence (AI) is based on computer programs that imitate human thinking and automate certain processes. Artificial intelligence has been studied in the medical field for over 50 years, but in recent years, its growth has been exponential. The field of cardiovascular imaging is particularly attractive since AI can guide non-experts in image acquisition, automate processes and measurements, guide diagnoses, detect findings not visible to the human eye, make opportunistic diagnoses of unexpected conditions in the index test, or identify patterns of association within a large amount of data as a source of hypothesis generation. In the field of cardiovascular prevention, AI has been used for diagnostic, prognostic, and therapeutic purposes in managing cardiovascular risk factors such as dyslipidemia and hypertension. While there are limitations to the use of AI, such as cost, accessibility, compatibility of programs, external validity of results in certain populations, and ethical-legal aspects such as data privacy, this technology is rapidly growing and is likely to revolutionize current medical practice.
Key words: Images; Cardiovascular prevention; Artificial intelligence; Machine learning
INTRODUCTION
The term artificial intelligence (AI) was coined in 1956 by John McCarthy, who ventured that "every aspect of learning or any other feature of intelligence can in principle be so precisely described that a machine can be made to simulate it". 1 In this line, we can understand AI as a branch of computer science that aims to create software programs capable of automating activities related to human thinking, such as decision making, problem solving, learning, and development of concepts and abstractions. 1,2,3 Machine learning (ML) is a subfield of AI, that develops algorithms capable of recognizing patterns in data, to solve certain tasks without being explicitly programmed to do so. Such algorithms do not require the constant intervention of a user to solve tasks in complex environments (autonomy) and can improve their performance based through the experience gained with new data (adaptability). 4,5 Deep Learning (DL) is a subfield of Machine Learning (ml) that involves the repetition of a processing block, arranged consecutively in layers to extract complex abstractions based on relatively simpler representations through a hierarchical learning process. 6,7,8 In general, DL models use Artificial Neural Networks (ANN) inspired by the way biological neural system works, such as the brain processes information. ANN consist of interconnected functional units called neurons that perform calculations and mathematical operations to propagate information. 7 A Deep Neural Network (DNN) is an ANN with multiple layers. A typical DNN consists of an input layer, one or more hidden layers, and an output layer; an output layer serves as input for the next one. 8,9,10 The performance of these methods in solving complex tasks in various fields of knowledge has led to their introduction in the medical field. Thus, the first clinical decision support systems used in Medicine, which relied on the depuration of medical knowledge databases and the formulation of robust decision rules, began to be replaced by data-driven systems, i.e., ML and DL models. 10 Implementing this type of solution has various impacts: 1) it provides professionals with a second opinion based on available data, which can improve diagnostic performance; b) it optimizes processes and workflows within the healthcare system; and c) patients benefit from an improvement in the quality of care. 9 AI has been utilized in Medicine for diagnostic, prognostic, and predictive purposes. It can automatically process various data modalities, including clinical, genetic, biomedical signals, and medical images, generated across different medical specialties. 10
AI in cardiovascular imaging
The use of AI in Medicine has been around for over half a century. In fact, as early as 1968, Earl Hunt wrote a review article on the subject applied to the field of Psychology. 11 The number of articles published on the subject has increased slowly since then, until about 5 years ago when the increase became exponential. In 2022 alone, nearly 39 000 articles were published on the subject, with approximately 1 in 3 articles devoted to imaging in Medicine.
The field of medical imaging is particularly attractive in this area, due to its exponential growth, complexity, and the limited availability of specialists to create and interpret such images in certain locations or at certain hours. The use of AI makes it possible to reduce acquisition and reading times, acquire and interpret images by untrained personnel, increase accuracy, and reduce the risk of diagnostic errors. On the other hand, AI has unique abilities such as detecting image features that may be difficult for humans to identify and finding unexpected patterns in an endless number of variables. These capabilities make AI a valuable tool for advancing new diagnoses and hypotheses. Combined with the ability to jointly evaluate a large amount of data beyond imaging, such as epidemiological, clinical, laboratory, genetic, and biometric data, the advances in science appear to be unpredictable. 12,13,14
Optimizing timing. Image acquisition by "inexperienced" personnel. Reducing variability
Image segmentation is a well-developed tool nowadays. AI can recognize and separate anatomical structures more quickly than a trained human. Once the segmentation is complete, the software can automatically measure diameters, volumes, displacements, flows, and other parameters of the test with an accuracy similar to or better than that of an expert. 15 (Figures 1 and 2).
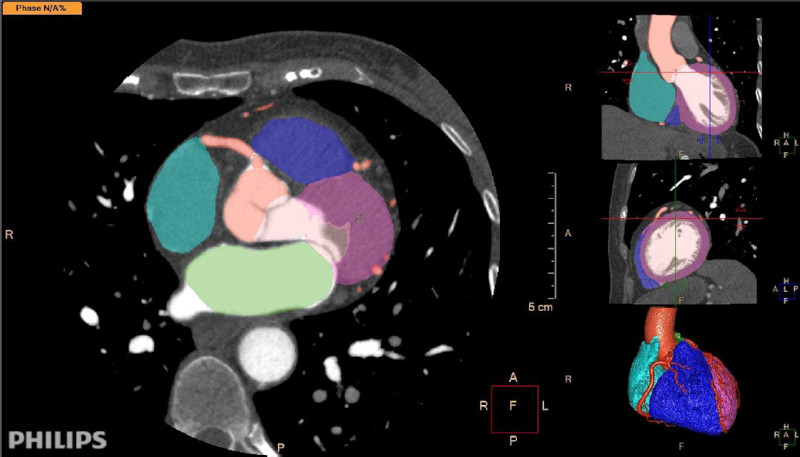
Fig. 1 Automated segmentation of a cardiovascular computed tomography scan with contrast agent. The software identifies the cardiac chambers, myocardium, coronary arteries, and ascending aorta. It provides segmentation of cardiac structures with different colors, which can be edited and corrected by professionals. Software: IntelliSpace Portal. Image and Information Management Software. Version 12.1. Philips Medical Systems Nederland B.V. Veenpluis 6, 5684 PC Best, The Netherlands
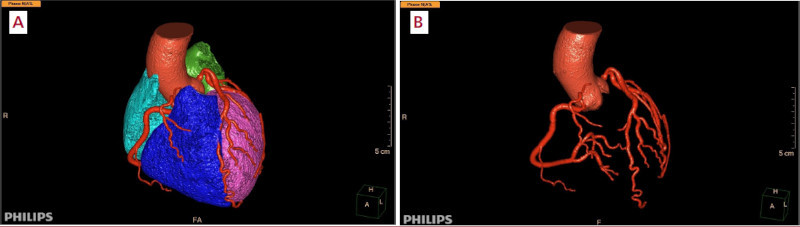
Fig. 2 A and B. Automatic cardiovascular reconstruction derived from Figure 1 (A) and with "removal" of all structures to visualize exclusively the aorta and coronary tree (B). Software: IntelliSpace Portal. Image and Information Management Software. Version 12.1. Philips Medical Systems Nederland B.V. Veenpluis 6, 5684 PC Best, The Netherlands.
Variability is one of many limitations of diagnostic methods. Results-based decision-making (diameters, ejection fraction, areas) can be a challenge for those interpreting imaging tests. AI is showing promising results in this regard due to its speed and reproducibility of measurements.
In echocardiography there are programs that can evaluate the quality of the acquired images, guide inexperienced operators to enhance their quality, automatically acquire them when the section detected is suitable for analysis, and perform automatic measurements. A study found that 8 nurses, without echocardiography training, could obtain AI-guided images from 30 patients each, with diagnostic quality comparable to that obtained by 5 registered cardiac sonographers. 16
The software can identify views and perform chambers, mass, volume, and strain measurements faster and more reproducibly than an experienced operator. Tsang et al. demonstrated the feasibility of measuring ventricular and atrial volumes, as well as ejection fraction, using 3D echocardiography with an adaptive algorithm. The automated measurements were comparable not only to manual measurements but also to those obtained by magnetic resonance imaging (MRI), with high reproducibility (zero variability when repeating the analysis on the same dataset). In addition, the average time per patient decreased from 144 ± 32 seconds (manual analysis) to 26 ± 2 seconds (automated analysis). 17
A prospective study utilized an AI training program to analyze diastolic function in accordance with ASE/EACVI 2016 guidelines. The program was shown to accurately classify diastolic dysfunction patterns with 99% agreement to the guidelines. 18
Estimating valvular heart disease severity can be difficult in some scenarios. Using AI, automated quantification of mitral regurgitation (MR) could be performed with high diagnostic accuracy (99.52% for normal mitral valve, 99.38% for mild MR, 99.31% for moderate MR and 99.59% for severe MR). 19 In patients with aortic stenosis, an AI system utilizing echocardiographic measurements, except for left ventricular outflow tract diameter and velocity, demonstrated higher accuracy in diagnosing severe aortic stenosis than the continuity equation, even in populations with ventricular dysfunction or low flow-low gradient aortic stenosis. 20 In cardiac MRI, planning and acquisition times, artifact correction, and automated measurements are advancing quickly, reducing the time needed for testing and analysis. 21 Artificial intelligence analysis was quicker (20 seconds per patient) and more accurate than human (13 min). 22 The authors estimate a 46% reduction in required trial sample size with the segmentation model based on AI with left ventricular ejection fraction as the endpoint.
In computed tomography (CT), AI is being evaluated to reduce radiation dose by optimizing image acquisition, converting non-contrast scans to contrast scans and vice versa so as to detect coronary artery calcification. 13
Improvement of diagnostic and prognostic processes: reduction of errors, opportunistic diagnoses
AI enables the identification of unexpected patterns or associations that link a condition or set of conditions to a specific finding or disease. Its use in echocardiography proved to be useful in differentiating between athlete's heart and hypertrophic cardiomyopathy, 23 between constrictive pericarditis and restrictive cardiomyopathy 24, and in the correct identification of patients with hypertrophic cardiomyopathy, amyloidosis, and pulmonary hypertension. 25
A multicenter study with cardiac MRI evaluated the precision of experts, trained junior physicians and ML to measure parameters of ventricular function, particularly ejection fraction, and the time required. Expert, trained junior, and ML precision were similar (coefficient of variation 6.1 [95% CI, 5.2%-7.1%], p = 0.2581; 8.3 [95% CI, 5.6%-10.4%], p = 0.3653; 8.8 [95% CI, 6.1%-11.1%], p = 0.8620, respectively). However, automated analysis was 186 times faster than humans (0.07 versus 13 minutes). 26
In Nuclear Medicine imaging, the use of AI demonstrated a superior performance in diagnosing obstructive coronary artery disease compared to the commonly used total perfusion deficit (similar specificity with higher sensitivity) and required less time for the analysis (0.5 seconds per patient). 27 The use of a Support Vector Machine algorithm demonstrated better performance than the analysis of ischemic changes, total perfusion deficit or ejection fraction changes, with an area under the ROC curve (AUC) significantly better than that of two independent expert operators. 28 In another study, when clinical variables were combined with information from stress testing and perfusion imaging, AI was superior to the existing isolated images, expert medical assessment or automated quantification in predicting events in 2619 patients followed up for more than 3 years. 29
Radiomics is another important aspect of clinically relevant information from medical imaging. Radiomics can identify tissue features that may indicate the type of tumor, characteristics of a lesion (acute or chronic), type of ventricular hypertrophy, or differences in myocardial tissue in response to risk factors by analyzing image characteristics not detectable by the human eye. 30,31,32
Mannil et al. demonstrated the ability of AI in detecting myocardial infarction on noncontrast computed tomography images, with a sensitivity of 86% and specificity of 81%, which was not possible by visual assessment of two readers with experience in cardiovascular imaging. 33
The utility of ML analysis in predicting cardiovascular events was demonstrated in the population of the CONFIRM study (10 030 patients) with 25 clinical parameters and 44 coronary computed tomography angiography parameters. ML analysis predicted 5-year mortality significantly better (AUC 0.79) than the Framingham risk score (AUC 0.61) or conventional coronary computed tomography angiography parameters (segment stenosis score, segment involvement score, or modified Duke index, AUC 0.64, 0.64, and 0.62, respectively). 34 Another analysis of 8844 patients from the same cohort revealed that a ML algorithm had greater prognostic accuracy than conventional segment analysis in predicting myocardial infarction or death at 3 years (AUC for ML 0.771, vs. AUC for conventional analysis between 0.685 and 0.701). 35
Similarly, investigators in the PARADIGM registry demonstrated a higher predictive value of a ML framework to identify individuals at risk for rapid progression of coronary atherosclerotic plaque. 36
In patients with newly diagnosed pulmonary hypertension, Dawes et al. used MRI to define a 3D model of right ventricular motion that more accurately predicted survival than conventional imaging and hemodynamic, functional, and clinical markers (AUC 0.73 vs. 0.60). 37
There are many examples of opportunistic diagnoses in the literature with the use of AI. Opportunistic findings are those that are unexpected and discovered on medical imaging tests performed for other reasons. 38 Pyrros et al. detected 5% of patients with diabetes using chest radiographs with acceptable diagnostic accuracy (AUC 0.77). 39 The detection of calcium plaques in the coronary arteries on a conventional chest CT scan (score >100) has been shown to have prognostic value for predicting 10-year mortality (HR 1.51; 95% CI 1.28-1.79); death, myocardial infarction, or stroke (HR 1.57; 95% CI 1.33-1.84); and death, myocardial infarction, stroke, or revascularization (HR 1.69; 95% CI 1.45-1.98) compared with no calcium. 40 Parameters indicating osteopenia or osteoporosis can be detected in chest or abdominal CT scans, 41 or in coronary artery calcium CT scans, 42 without additional cost or radiation exposure to patients. Similarly, detection of atheromatous plaques, fatty liver disease, sarcopenia, and visceral adipose tissue can identify patients at risk of metabolic syndrome and/ or long-term events such as death, cardiovascular events, and fractures. Early detection of these risks could be cost-effective. 43,44 DL-based models have been shown to improve the speed and accuracy of emphysema detection. 45
Implementation of AI in the field of cardiovascular prevention
Multiple AI-based techniques have been implemented in different scenarios associated with cardiovascular prevention. One of them is the diagnosis of familial hypercholesterolemia (FH), the most common genetic disorder of lipid metabolism. Familial hypercholesterolemia results in exposure to very high levels of low-density lipoprotein cholesterol (LDL-C) throughout lifetime, significantly increasing the risk of cardiovascular events if left untreated. 46 The clinical diagnosis of FH (clinical scores) can be established by collecting clinical and laboratory (LDL-C) features. The diagnosis of FH can be confirmed genetically by identifying heterozygous or biallelic phenotypes, especially in genes LDLR, APOB or PCSK9. 47 However, AI techniques have the potential to improve the identification of patients with FH. In this context, several studies have demonstrated that AI-based models have an excellent predict value to detect FH cases confirmed by genetic testing. 48,49,50,51 Furthermore, that predictive value performed better than traditional methods based on risk scores, such as the Dutch Lipid Clinic Network criteria.
Another scenario in which AI was evaluated is the calculation of LDL-C. Standard practice estimates LDL-C using the Friedewald formula. 52 However, this equation is inaccurate when triglycerides are elevated or LDL-C values are very low. 53 To improve accuracy, other formulas have been developed, such as the Martin-Hopkins formula or the Sampson equation. 54,55 Many studies have evaluated different AI-based techniques to estimate LDL-C. 56,57,58,59,60,61 In this case, the AI-based algorithms for estimating LDL-C performed similarly or better than the conventional Friedewald formula or the more contemporary Martin-Hopkins or Sampson equations.
The barriers associated with the use of statins is another field where AI has been evaluated. Statins intolerance represents a clinical challenge, and its prevalence is frequently overestimated. 62 Importantly, the nocebo effect could play a key role in this significant public health issue. 63 A recent study assessed AI-based techniques for analyzing large amounts of publicly available social media data to generate insights into people's perceptions about statins. 64 The analysis revealed predominantly neutral to negative sentiment in the discussions analyzed, with 30.8%, 66.6% and 2.6% reporting negative, neutral or positive sentiments, respectively. Sarraju et al. developed a DL-based model to identify statin nonuse and reasons for statin nonuse using unstructured electronic medical records from a large cohort of patients with atherosclerotic cardiovascular disease. 65 The model identified key patient-level reasons (side effects, patient preference) and clinician-level reasons (guideline- discordant practices) for statin nonuse. Another study used a DL-based algorithm in patients with diabetes. 66 Again, this algorithm categorized the reasons for statin nonuse based on a large dataset of electronic medical records, including patient reasons (side effects and hesitancy to use statins), physician reasons (guideline‐discordant practice), and system reasons (clinical inertia). Understanding the barriers that patients and physicians face in using statins can help reduce clinical inertia and improve treatment adherence.
AI has also been evaluated in hypertension. According to some reports, AI-based systems could help continuously monitor blood pressure using wearable technologies. 67 For example, blood pressure could be estimated from a photoplethysmography signal obtained from a smartphone or a smartwatch using DL. Furthermore, ML algorithms can help make early diagnosis of hypertension 68,69,70 and even of secondary hypertension. 71 In addition, ML algorithms accurately predict ambulatory blood pressure levels after treatment initiation. This could assist clinicians in personalizing antihypertensive treatment. 72,73
Several cardiovascular risk functions or scores were developed based on large epidemiological studies. Usually, these scores can be calculated based on a few variables that are easy to obtain in the office. Although these tools are very useful in clinical practice, they have limitations related to calibration and discrimination ability. 74 In this context, many studies have incorporated AI/ML-based algorithms to optimize cardiovascular risk prediction. These studies, conducted in different regions worldwide, evaluated multiple algorithms utilizing different AI techniques. These algorithms included more variables than those typically used to stratify cardiovascular risk. 75,76,77,78 In general, these models, which utilized data from thousands of patients, have shown excellent predictive ability. In some cases, they have even outperformed traditional scores like the Framingham risk score. 75 Another AI-based model that considered the presence of subclinical atheromatosis (qualitative analysis of atherosclerotic plaques by CT) had significant prognostic value for major cardiovascular events, and showed additional value over clinical risk factors, coronary artery calcium score, or traditional methods for evaluating CT scan images. 79
Challenges and limitations
The usefulness of AI in Medicine is undeniable, and its implementation is rapidly expanding. However, it is not free of limitations.
Firstly, many of the programs solve some specific aspects (such as automated segmentation and/ or quantification), but not others relevant to image analysis. The development cost of these programs is very high, which limits their availability.
Another important aspect is applicability. Although many AI-based results are applicable in clinical practice, some findings may lead to conclusions with limited clinical value, especially in the current stage of Medicine. This may lead professionals to disregard the results because they deviate from the usual clinical findings of their practice. Additionally, the development of the program may have been biased by the selected population, which could limit its generalizability to other populations with different characteristics. This, in turn, limits the external validity of the program.
Similarly, some AI programs based on DL create models that are difficult or impossible to interpret due to their complexity, resulting in what is known as the "black box" effect. Professionals who must interpret the results may find it difficult to accept or reject them because they cannot understand how the model used to determine the results was constructed. This raises clinical, ethical and even legal issues when assessing responsibility in the decision-making process. 80,81
As with previous analysis programs, it is important to obtain consistent results across different software programs to ensure that imaging tests can be analyzed using programs from different brands or generic analysis software.
And. finally, data privacy is another crucial element when considering this type of tool.
CONCLUSIONES
AI enables image acquisition by non-expert person nel, automated image analysis with a similar level of correlation to experts, but with greater speed and accuracy. AI can analyze image features to detect as pects that are not identifiable by the human eye. This can guide the diagnosis of new diseases or prognosis. The combination of these variables, along with other available data, enables a diagnostic and prognostic ap proach that is superior to imaging alone for both clini cal practice and research studies. In the field of car diovascular prevention, AI has enabled more accurate diagnosis of FH, calculation of LDL-C, identification of barriers to statin use, early diagnosis of hypertension, or prediction of clinical events superior to traditional scores. This new technology is not free of biases and limitations, but it has undoubtedly been incorporated into everyday practice and is likely to revolutionize medical activity, at least as we know it today.
REFERENCES
1. McCarthy J, Minsky ML, Rochester N, Shannon CE. A Proposal for the Dartmouth Summer Research Project on Artificial Intelligence, August 31, 1955. AI Mag. 2006;27:12. https://doi.org/10.1609/aimag.v27i4.1904 [ Links ]
2. Bellman R. An Introduction to Artificial Intelligence: Can Computers Think? Boyd & Fraser Publishing Company; 1978. 168 p. [ Links ]
3. Russell S, Norvig P. Artificial intelligence. A modern approach. 3rd ed. New Jersey: Pearson Education; 2010. [ Links ]
4. Tom M. Mitchell. Machine Learning. New York: McGraw-Hill; 1997. 414 p. (McGraw-Hill series in computer science). [ Links ]
5. Kevin P Murphy. Machine Learning. A Probabilistic Perspective. Cambridge, Massachusetts: The MIT Press; 2012. [ Links ]
6. LeCun Y, Bengio Y, Hinton G. Deep learning. Nature. 2015 May 28;521(7553):436-44. https://doi.org/10.1038/nature14539 [ Links ]
7. Ian Goodfellow, Yoshua Bengio, Aaron Courville. Deep Learning. The MIT Press; 2016. [ Links ]
8. Mesko Bertalan. A Guide to Artificial Intelligence in Health Care. Victoria, British Columbia: Leanpub; 2017. [ Links ]
9. Topol EJ. High-performance medicine: the convergence of human and artificial intelligence. Nat Med. 2019;25:44-56. https://doi.org/10.1038/s41591-018-0300-7 [ Links ]
10. Yu KH, Beam AL, Kohane IS. Artificial intelligence in healthcare. Nat Biomed Eng. 2018;2:719-31. https://doi.org/10.1038/s41551-018-0305-z [ Links ]
11. Hunt E. Computer simulation: artificial intelligence studies and their relevance to psychology. Annu Rev Psychol. 1968;19:135-68. https://doi.org/10.1146/annurev.ps.19.020168.001031 [ Links ]
12. Haq IU, Haq I, Xu B. Artificial intelligence in personalized cardiovascular medicine and cardiovascular imaging. Cardiovasc Diagn Ther. 2021;11:911-23. https://doi.org/10.21037/cdt.2020.03.09 [ Links ]
13. Badano LP, Keller DM, Muraru D, Torlasco C, Parati G. Artificial intelligence and cardiovascular imaging: A win-win combination. Anatol J Cardiol. 2020;24:214-23. https://doi.org/10.14744/AnatolJCardiol.2020.94491 [ Links ]
14. Seetharam K, Min JK. Artificial Intelligence and Machine Learning in Cardiovascular Imaging. Methodist Debakey Cardiovasc J. 2020;16:263-71. https://doi.org/10.14797/mdcj-16-4-263 [ Links ]
15. Dey D, Slomka PJ, Leeson P, Comaniciu D, Shrestha S, Sengupta PP, et al. Artificial Intelligence in Cardiovascular Imaging: JACC State-of-the-Art Review. J Am Coll Cardiol. 2019;73:1317-35. https://doi.org/10.1016/j.jacc.2018.12.054 [ Links ]
16. Narang A, Bae R, Hong H, Thomas Y, Surette S, Cadieu C, et al. Utility of a Deep-Learning Algorithm to Guide Novices to Acquire Echocardiograms for Limited Diagnostic Use. JAMA Cardiol. 2021;6:624-32. https://doi.org/10.1001/jamacardio.2021.0185 [ Links ]
17. Tsang W, Salgo IS, Medvedofsky D, Takeuchi M, Prater D, Weinert L, et al. Transthoracic 3D Echocardiographic Left Heart Chamber Quantification Using an Automated Adaptive Analytics Algorithm. JACC Cardiovasc Imaging. 2016;9:769-82. https://doi.org/10.1016/j.jcmg.2015.12.020 [ Links ]
18. Jiang R, Yeung D, Behnami D, Jue J, Tsang M, Gin K, et al. Machine Learning to facilitate assessment of diastolic function by echocardiography. Can J Cardiol. 2019;35:S4-5. https://doi.org/10.1016/j.cjca.2019.07.060 [ Links ]
19. Moghaddasi H, Nourian S. Automatic assessment of mitral regurgitation severity based on extensive textural features on 2D echocardiography videos. Comput Biol Med. 2016;73:47-55. https://doi.org/10.1016/j.compbiomed.2016.03.026 [ Links ]
20. Playford D, Bordin E, Mohamad R, Stewart S, Strange G. Enhanced Diagnosis of Severe Aortic Stenosis Using Artificial Intelligence: A Proof-of-Concept Study of 530,871 Echocardiograms. JACC Cardiovasc Imaging. 2020;13:1087-90. https://doi.org/10.1016/j.jcmg.2019.10.013 [ Links ]
21. Leiner T, Rueckert D, Suinesiaputra A, Baeβler B, Nezafat R, Išgum I, et al. Machine learning in cardiovascular magnetic resonance: basic concepts and applications. J Cardiovasc Magn Reson. 2019;21:61. https://doi.org/10.1186/s12968-019-0575-y [ Links ]
22. Davies RH, Augusto JB, Bhuva A, Xue H, Treibel TA, Ye Y, et al. Precision measurement of cardiac structure and function in cardiovascular magnetic resonance using machine learning. J Cardiovasc Magn Reson. 2022;24:16. https://doi.org/10.1186/s12968-022-00846-4 [ Links ]
23. Narula S, Shameer K, Salem Omar AM, Dudley JT, Sengupta PP. Machine-Learning Algorithms to Automate Morphological and Functional Assessments in 2D Echocardiography. J Am Coll Cardiol. 2016;68:2287-95. https://doi.org/10.1016/j.jacc.2016.08.062 [ Links ]
24. Sengupta PP, Huang YM, Bansal M, Ashrafi A, Fisher M, Shameer K, et al. Cognitive Machine-Learning Algorithm for Cardiac Imaging: A Pilot Study for Differentiating Constrictive Pericarditis From Restrictive Cardiomyopathy. Circ Cardiovasc Imaging. 2016;9:e004330. https://doi.org/10.1161/CIRCIMAGING.115.004330 [ Links ]
25. Zhang J, Gajjala S, Agrawal P, Tison GH, Hallock LA, Beussink- Nelson L, et al. Fully Automated Echocardiogram Interpretation in Clinical Practice. Circulation. 2018;138:1623-35. https://doi.org/10.1161/CIRCULATIONAHA.118.034338 [ Links ]
26. Bhuva AN, Bai W, Lau C, Davies RH, Ye Y, Bulluck H, et al. A Multicenter, Scan-Rescan, Human and Machine Learning CMR Study to Test Generalizability and Precision in Imaging Biomarker Analysis. Circ Cardiovasc Imaging. 2019;12:e009214. https://doi.org/10.1161/CIRCIMAGING.119.009214 [ Links ]
27. Betancur J, Commandeur F, Motlagh M, Sharir T, Einstein AJ, Bokhari S, et al. Deep Learning for Prediction of Obstructive Disease From Fast Myocardial Perfusion SPECT: A Multicenter Study. JACC Cardiovasc Imaging. 2018;11:1654-63. https://doi.org/10.1016/j.jcmg.2018.01.020 [ Links ]
28. Arsanjani R, Xu Y, Dey D, Fish M, Dorbala S, Hayes S, et al. Improved accuracy of myocardial perfusion SPECT for the detection of coronary artery disease using a support vector machine algorithm. J Nucl Med. 2013;54:549-55. https://doi.org/10.2967/jnumed.112.111542 [ Links ]
29. Betancur J, Otaki Y, Motwani M, Fish MB, Lemley M, Dey D, et al. Prognostic Value of Combined Clinical and Myocardial Perfusion Imaging Data Using Machine Learning. JACC Cardiovasc Imaging. 2018;11:1000-9. https://doi.org/10.1016/j.jcmg.2017.07.024 [ Links ]
30. Raisi-Estabragh Z, Izquierdo C, Campello VM, Martin-Isla C, Jaggi A, Harvey NC, et al. Cardiac magnetic resonance radiomics: basic principles and clinical perspectives. Eur Heart J Cardiovasc Imaging. 2020;21:349-56. https://doi.org/10.1093/ehjci/jeaa028 [ Links ]
31. Schofield R, Ganeshan B, Fontana M, Nasis A, Castelletti S, Rosmini S, et al. Texture analysis of cardiovascular magnetic resonance cine images differentiates aetiologies of left ventricular hypertrophy. Clin Radiol. 2019;74:140-9. https://doi.org/10.1016/j.crad.2018.09.016 [ Links ]
32. Cetin I, Raisi-Estabragh Z, Petersen SE, Napel S, Piechnik SK, Neubauer S, et al. Radiomics Signatures of Cardiovascular Risk Factors in Cardiac MRI: Results From the UK Biobank. Front Cardiovasc Med. 2020;7:591368. https://doi.org/10.3389/fcvm.2020.591368 [ Links ]
33. Mannil M, von Spiczak J, Manka R, Alkadhi H. Texture Analysis and Machine Learning for Detecting Myocardial Infarction in Noncontrast Low-Dose Computed Tomography: Unveiling the Invisible. Invest Radiol. 2018;53:338-43. https://doi.org/10.1097/RLI.0000000000000448 [ Links ]
34. Motwani M, Dey D, Berman DS, Germano G, Achenbach S, Al- Mallah MH, et al. Machine learning for prediction of all-cause mortality in patients with suspected coronary artery disease: a 5-year multicentre prospective registry analysis. Eur Heart J. 2017;38:500- 7. https://doi.org/10.1093/eurheartj/ehw188 [ Links ]
35. van Rosendael AR, Maliakal G, Kolli KK, Beecy A, Al'Aref SJ, Dwivedi A, et al. Maximization of the usage of coronary CTA derived plaque information using a machine learning based algorithm to improve risk stratification; insights from the CONFIRM registry. J Cardiovasc Comput Tomogr. 2018;12:204-9. https://doi.org/10.1016/j.jcct.2018.04.011 [ Links ]
36. Han D, Kolli KK, Al'Aref SJ, Baskaran L, van Rosendael AR, Gransar H, et al. Machine Learning Framework to Identify Individuals at Risk of Rapid Progression of Coronary Atherosclerosis: From the PARADIGM Registry. J Am Heart Assoc. 2020;9:e013958. https://doi.org/10.1161/JAHA.119.013958 [ Links ]
37. Dawes TJW, de Marvao A, Shi W, Fletcher T, Watson GMJ, Wharton J, et al. Machine Learning of Three-dimensional Right Ventricular Motion Enables Outcome Prediction in Pulmonary Hypertension: A Cardiac MR Imaging Study. Radiology. 2017;283:381-90. https://doi.org/10.1148/radiol.2016161315 [ Links ]
38. Pickhardt PJ, Summers RM, Garrett JW, Krishnaraj A, Agarwal S, Dreyer KJ, et al. Opportunistic Screening: Radiology Scientific Expert Panel. Radiology. 2023;307:e222044. https://doi.org/10.1148/radiol.222044 [ Links ]
39. Pyrros A, Borstelmann SM, Mantravadi R, Zaiman Z, Thomas K, Price B, et al. Opportunistic detection of type 2 diabetes using deep learning from frontal chest radiographs. Nat Commun. 2023;14:4039. https://doi.org/10.1038/s41467-023-39631-x [ Links ]
40. Peng AW, Dudum R, Jain SS, Maron DJ, Patel BN, Khandwala N, et al. Association of Coronary Artery Calcium Detected by Routine Ungated CT Imaging With Cardiovascular Outcomes. J Am Coll Cardiol. 2023;82:1192-202. https://doi.org/10.1016/j.jacc.2023.06.040 [ Links ]
41. Yang J, Liao M, Wang Y, Chen L, He L, Ji Y, et al. Opportunistic osteoporosis screening using chest CT with artificial intelligence. Osteoporos Int. 2022;33:2547-61. https://doi.org/10.1007/s00198-022-06491-y [ Links ]
42. Naghavi M, Atlas K, Jaberzadeh A, Zhang C, Manubolu V, Li D, et al. Validation of Opportunistic Artificial Intelligence-Based Bone Mineral Density Measurements in Coronary Artery Calcium Scans. J Am Coll Radiol. 2023:S1546-1440(23)00405-2. https://doi.org/10.1016/j.jacr.2023.05.006 [ Links ]
43. Pickhardt PJ, Correale L, Hassan C. AI-based opportunistic CT screening of incidental cardiovascular disease, osteoporosis, and sarcopenia: cost-effectiveness analysis. Abdom Radiol (NY). 2023;48:1181-98. https://doi.org/10.1007/s00261-023-03800-9 [ Links ]
44. Lee MH, Zea R, Garrett JW, Graffy PM, Summers RM, Pickhardt PJ. Abdominal CT Body Composition Thresholds Using Automated AI Tools for Predicting 10-year Adverse Outcomes. Radiology. 2023;306:e220574. https://doi.org/10.1148/radiol.220574 [ Links ]
45. Fuhrman J, Yip R, Zhu Y, Jirapatnakul AC, Li F, Henschke CI, et al. Evaluation of emphysema on thoracic low-dose CTs through attention-based multiple instance deep learning. Sci Rep. 2023;13:1187. https://doi.org/10.1038/s41598-023-27549-9 [ Links ]
46. Kallapur A, Sallam T. Pharmacotherapy in familial hypercholesterolemia - Current state and emerging paradigms. Trends Cardiovasc Med. 2023;33:170-9. https://doi.org/10.1016/j.tcm.2021.12.011 [ Links ]
47. Berberich AJ, Hegele RA. The complex molecular genetics of familial hypercholesterolaemia. Nat Rev Cardiol. 2019;16:9-20. https://doi.org/10.1038/s41569-018-0052-6 [ Links ]
48. Hesse R, Raal FJ, Blom DJ, George JA. Familial Hypercholesterolemia Identification by Machine Learning Using Lipid Profile Data Performs as Well as Clinical Diagnostic Criteria. Circ Genom Precis Med. 2022;15:e003324. https://doi.org/10.1161/CIRCGEN.121.003324 [ Links ]
49. Pina A, Helgadottir S, Mancina RM, Pavanello C, Pirazzi C, Montalcini T, et al. Virtual genetic diagnosis for familial hypercholesterolemia powered by machine learning. Eur J Prev Cardiol. 2020;27:1639-46. https://doi.org/10.1177/2047487319898951 [ Links ]
50. Nolde JM, Pang J, Chan DC, Ward NC, Mian A, Schlaich MP, et al. Neural Network Modelling for Predicting Gene Variants Causative of Familial Hypercholesterolaemia in the Clinic. Heart Lung Circ. 2023;32:44-5. https://doi.org/10.1016/j.hlc.2023.04.003 [ Links ]
51. Banda JM, Sarraju A, Abbasi F, Parizo J, Pariani M, Ison H, et al. Finding missed cases of familial hypercholesterolemia in health systems using machine learning. NPJ Digit Med. 2019;2:23. https://doi.org/10.1038/s41746-019-0101-5 [ Links ]
52. Friedewald WT, Levy RI, Fredrickson DS. Estimation of the concentration of low-density lipoprotein cholesterol in plasma, without use of the preparative ultracentrifuge. Clin Chem. 1972;18:499-502 [ Links ]
53. Rossouw HM, Nagel SE, Pillay TS. Comparability of 11 different equations for estimating LDL cholesterol on different analysers. Clin Chem Lab Med. 2021;59:1930-43. https://doi.org/10.1515/cclm-2021-0747 [ Links ]
54. Martin SS, Blaha MJ, Elshazly MB, Toth PP, Kwiterovich PO, Blumenthal RS, et al. Comparison of a novel method vs the Friedewald equation for estimating low-density lipoprotein cholesterol levels from the standard lipid profile. JAMA. 2013;310:2061-8. https://doi.org/10.1001/jama.2013.280532 [ Links ]
55. Sampson M, Ling C, Sun Q, Harb R, Ashmaig M, Warnick R, et al. A New Equation for Calculation of Low-Density Lipoprotein Cholesterol in Patients With Normolipidemia and/or Hypertriglyceridemia. JAMA Cardiol. 2020;5:540-8. https://doi.org/10.1001/jamacardio.2020.0013 [ Links ]
56. Oh GC, Ko T, Kim JH, Lee MH, Choi SW, Bae YS, et al. Estimation of low-density lipoprotein cholesterol levels using machine learning. Int J Cardiol. 2022;352:144-9. https://doi.org/10.1016/j.ijcard.2022.01.029 [ Links ]
57. Barakett-Hamade V, Ghayad JP, Mchantaf G, Sleilaty G. Is Machine Learning-derived Low-Density Lipoprotein Cholesterol estimation more reliable than standard closed form equations? Insights from a laboratory database by comparison with a direct homogeneous assay. Clin Chim Acta. 2021;519:220-6. https://doi.org/10.1016/j.cca.2021.05.008 [ Links ]
58. Singh G, Hussain Y, Xu Z, Sholle E, Michalak K, Dolan K, et al. Comparing a novel machine learning method to the Friedewald formula and Martin-Hopkins equation for low-density lipoprotein estimation. PLoS One. 2020;15:e0239934. https://doi.org/10.1371/journal.pone.0239934 [ Links ]
59. Ghayad JP, Barakett-Hamadé V, Sleilaty G. Prospective Validation of a Machine Learning Model for Low-Density Lipoprotein Cholesterol Estimation. Lab Med. 2022;53:629-35. https://doi.org/10.1093/labmed/lmac049 [ Links ]
60. Hidekazu I, Nagasawa H, Yamamoto Y, Doi H, Saito M, Ishihara Y, et al. Dataset dependency of low-density lipoprotein-cholesterol estimation by machine learning. Ann Clin Biochem. 2023;60:396- 405. https://doi.org/10.1177/00045632231180408 [ Links ]
61. Kwon YJ, Lee H, Baik SJ, Chang HJ, Lee JW. Comparison of a Machine Learning Method and Various Equations for Estimating Low-Density Lipoprotein Cholesterol in Korean Populations. Front Cardiovasc Med. 2022;9:824574. https://doi.org/10.3389/fcvm.2022.824574 [ Links ]
62. Bytyçi I, Penson PE, Mikhailidis DP, Wong ND, Hernandez AV, Sahebkar A, et al. Prevalence of statin intolerance: a meta-analysis. Eur Heart J. 2022;43:3213-23. https://doi.org/10.1093/eurheartj/ehac015 [ Links ]
63. Penson PE, Mancini GBJ, Toth PP, Martin SS, Watts GF, Sahebkar A,et al. Lipid and Blood Pressure Meta-Analysis Collaboration (LBPMC) Group & International Lipid Expert Panel (ILEP). Introducing the 'Drucebo' effect in statin therapy: a systematic review of studies comparing reported rates of statin-associated muscle symptoms, under blinded and open-label conditions. J Cachexia Sarcopenia Muscle. 2018;9:1023-33. https://doi.org/10.1002/jcsm.12344s [ Links ]
64. Somani S, van Buchem MM, Sarraju A, Hernandez-Boussard T, Rodriguez F. Artificial Intelligence-Enabled Analysis of Statin-Related Topics and Sentiments on Social Media. JAMA Netw Open. 2023;6:e239747. https://doi.org/10.1001/jamanetworkopen.2023.9747 [ Links ]
65. Sarraju A, Coquet J, Zammit A, Chan A, Ngo S, Hernandez- Boussard T, et al. Using deep learning-based natural language processing to identify reasons for statin nonuse in patients with atherosclerotic cardiovascular disease. Commun Med (Lond). 2022;2:88. https://doi.org/10.1038/s43856-022-00157-w [ Links ]
66. Sarraju A, Zammit A, Ngo S, Witting C, Hernandez-Boussard T, Rodriguez F. Identifying Reasons for Statin Nonuse in Patients With Diabetes Using Deep Learning of Electronic Health Records. J Am Heart Assoc. 2023;12:e028120. https://doi.org/10.1161/JAHA.122.028120 [ Links ]
67. Visco V, Izzo C, Mancusi C, Rispoli A, Tedeschi M, Virtuoso N, et al. Artificial Intelligence in Hypertension Management: An Ace up Your Sleeve. J Cardiovasc Dev Dis. 2023;10:74. https://doi.org/10.3390/jcdd10020074 [ Links ]
68. Ye C, Fu T, Hao S, Zhang Y, Wang O, Jin B, et al. Prediction of Incident Hypertension Within the Next Year: Prospective Study Using Statewide Electronic Health Records and Machine Learning. J Med Internet Res. 2018;20:e22. https://doi.org/10.2196/jmir.9268 [ Links ]
69. Kanegae H, Suzuki K, Fukatani K, Ito T, Harada N, Kario K. Highly precise risk prediction model for new-onset hypertension using artificial intelligence techniques. J Clin Hypertens (Greenwich). 2020;22:445-50. https://doi.org/10.1111/jch.13759 [ Links ]
70. Golino HF, Amaral LS, Duarte SF, Gomes CM, Soares Tde J, Dos Reis LA, et al. Predicting increased blood pressure using machine learning. J Obes. 2014;2014:637635. https://doi.org/10.1155/2014/637635 [ Links ]
71. Wu L, Huang L, Li M, Xiong Z, Liu D, Liu Y, et al. Differential diagnosis of secondary hypertension based on deep learning. Artif Intell Med. 2023;141:102554. https://doi.org/10.1016/j.artmed.2023.102554 [ Links ]
72. Hae H, Kang SJ, Kim TO, Lee PH, Lee SW, Kim YH, et al. Machine Learning-Based prediction of Post-Treatment ambulatory blood pressure in patients with hypertension. Blood Press. 2023;32:2209674. https://doi.org/10.1080/08037051.2023.2209674 [ Links ]
73. Ye X, Zeng QT, Facelli JC, Brixner DI, Conway M, Bray BE. Predicting Optimal Hypertension Treatment Pathways Using Recurrent Neural Networks. Int J Med Inform. 2020;139:104122. https://doi.org/10.1016/j.ijmedinf.2020.104122 [ Links ]
74. Baena-Diez JM, Ramor R, Marrugat J. Predictive Value of Cardiovascular Risk Functions: Limitations and Future Potential. Rev Esp Cardiol. 2009;(9 Suppl):4B-13B. https://doi.org/10.1016/S1131-3587(09)71500-6 [ Links ]
75. Weng SF, Reps J, Kai J, Garibaldi JM, Qureshi N. Can machinelearning improve cardiovascular risk prediction using routine clinical data? PLoS One. 2017;12:e0174944. https://doi.org/10.1371/journal.pone.0174944 [ Links ]
76. Alaa AM, Bolton T, Di Angelantonio E, Rudd JHF, van der Schaar M. Cardiovascular disease risk prediction using automated machine learning: A prospective study of 423,604 UK Biobank participants. PLoS One. 2019;14:e0213653. https://doi.org/10.1371/journal.pone.0213653 [ Links ]
77. Kim JOR, Jeong YS, Kim JH, Lee JW, Park D, Kim HS. Machine Learning-Based Cardiovascular Disease Prediction Model: A Cohort Study on the Korean National Health Insurance Service Health Screening Database. Diagnostics (Basel). 2021;11:943. https://doi.org/10.3390/diagnostics11060943 [ Links ]
78. Mehrabani-Zeinabad K, Feizi A, Sadeghi M, Roohafza H, Talaei M, Sarrafzadegan N. Cardiovascular disease incidence prediction by machine learning and statistical techniques: a 16-year cohort study from eastern Mediterranean region. BMC Med Inform Decis Mak. 2023;23:72. https://doi.org/10.1186/s12911-023-02169-5 [ Links ]
79. Nurmohamed NS, Bom MJ, Jukema RA, de Groot RJ, Driessen RS, van Diemen PA, et al. AI-Guided Quantitative Plaque Staging Predicts Long-Term Cardiovascular Outcomes in Patients at Risk for Atherosclerotic CVD. JACC Cardiovasc Imaging. 2023:S1936- 878X(23)00277-2. https://doi.org/10.1016/j.jcmg.2023.05.020 [ Links ]
80. Lang M, Bernier A, Knoppers BM. Artificial Intelligence in Cardiovascular Imaging: "Unexplainable" Legal and Ethical Challenges? Can J Cardiol. 2022;38:225-33. https://doi.org/10.1016/j.cjca.2021.10.009 [ Links ]
81. Bernard O, Lalande A, Zotti C, Cervenansky F, Yang X, Heng PA, et al. Deep Learning Techniques for Automatic MRI Cardiac Multi-Structures Segmentation and Diagnosis: Is the Problem Solved? IEEE Trans Med Imaging. 2018;37:2514-25. https://doi.org/10.1109/TMI.2018.2837502 [ Links ]
Received: January 03, 2024; Accepted: January 24, 2024